Everything You Need to Know About Multimodal AI: What It Is, How It Works, Its Benefits, and More
Learn everything you need to know about Multimodal AI or Multimodal GenAI: What it is, how it works, its benefits and more.
- Jul 12 2024
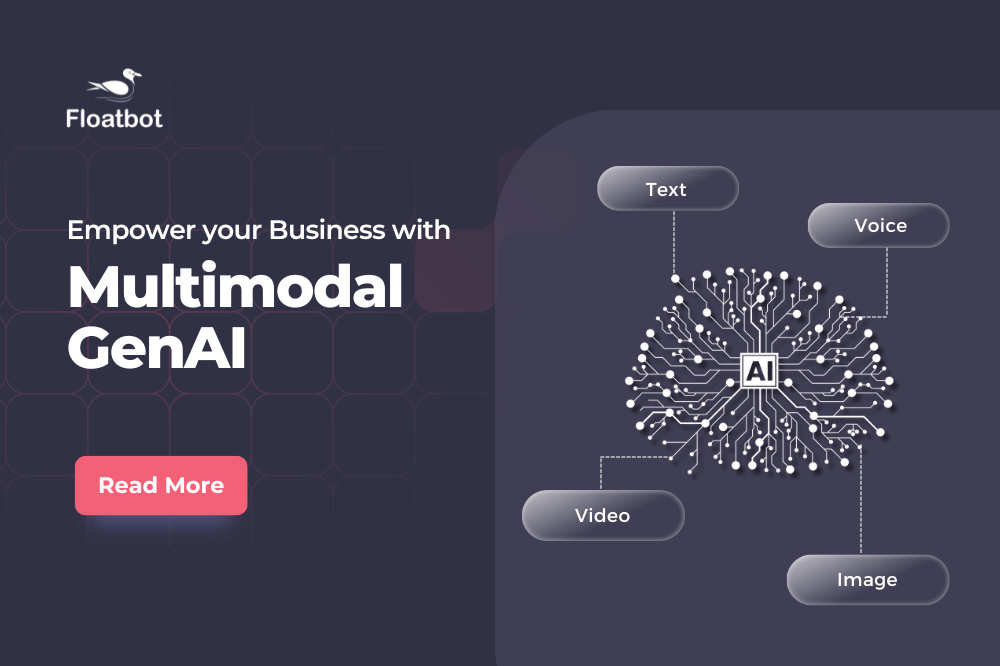
What is Multi Modal AI?
Multi modal AI is a type of artificial intelligence that utilizes multiple data sources to predict accurate response based on the user input. Multi modal AI have multiple modes of communication, you can prompt a model with any input and can generate any content type. Multi modal AI models can be trained on text, audio, images, videos and multiple other numerical data sets. It uses numerous forms of data to better interpret context of the query. The AI model is trained on multiple sources/modalities to make the predictions, in short combining information from different sources such as text, image, audio and video to build complete and accurate understanding of underlying data.
Multi modal mean one or more of the following
- Input and output are of different modalities
- Inputs are multi modals
- Outputs are multimodals
Multi Modal Vs Single Modal AI
The basic difference between multi modal to single modal AI is the data, single modal is trained on single type of data whereas multi modal is trained on various types of data from multiple sources. For a long time, AI models were operated on one data mode – text, image or audio making it limited and cannot handle multiple data types simultaneously nor can generate data with different modalities.
Multi-modal AI is versatile and is able to understand and generate multiple data types, on the other hand single-modal AI cannot handle data diversity.
How Multi-modal AI works?
Multi-modal AI depends on three modules to process different types of data formats. Input module, fusion module and output module. Input module comprises of neural networks that are trained to recognize and process various types of data, from text to images. Fusion module processes the data such as words, phrases, images etc. Each data type is pre-processed in its own way.
Multimodal works on 3 modules that helps it in understanding multiple data modes like text, images, audio, video etc., Multi-modal AI system consist of multiple technologies across its stack such as
Input module
This module comprises of neural networks that receive and process different types of data such as text, image, speech etc. This module is capable of handling diverse inputs. It is responsible for receiving and processing data from various sources.
It essentially consists of unimodal neural networks which are trained to handle specific types of data. For instance, one network might be an expert in understanding text (natural language processing), while another might be an expert at recognizing objects in images (computer vision).
Each data type goes through its own preprocessing steps within the corresponding neural network. This preprocessing might involve breaking down text into words or phrases (tokenization), extracting features from images (edge detection), and so on.
Fusion Module
The fusion module receives the preprocessed data (features) extracted from each modality by the input module. These data can be key terms from text, numerical representations, or object outlines from images. The fusion module combines this information. Fusion module is the integration point, where information from various data modalities is combined to create a richer understanding. There are several ways to perform fusion, depending on the complexity of the data, here are three common techniques:
- Early Fusion
- Intermediate Fusion
- Late Fusion
Output Module
Output module is responsible for generating a response. In output module the processed information is transformed into a response or action.
Here’s a breakdown of its role.
Understanding the goal: The output module needs to consider the purpose of the entire multimodal AI system. Is it designed to make predictions, generate creative text formats, answer questions, or control a robot? This goal dictates the format of the output.
- Tailoring the Response: Depending on the task, the output can take many forms. For instance:
- Textual Response: The system might generate summaries of information, translate languages, or write different kinds of creative content.
- Visual Output: The AI could create images, translate text descriptions into images, or manipulate existing visuals.
- Actionable Decisions: In some cases, the output might be a control signal for a robot or a recommendation for a human user.
- Presentation Matters: The way the output is presented is also important. The module might need to format text for readability, adjust the style of an image for its intended use, or prioritize the most relevant recommendations for a user.
Here is an example in action:
Here is an example of how the output module might function:
- A multimodal customer service AI: This system might analyze a customer's text message (input module), combine it with past purchase history and sentiment analysis of voice recordings (fusion module), and then generate a personalized response dedicated to the customer's needs (output module).
What is Multimodal Generative AI?
Multimodal Generative AI is an AI system that is capable of understanding, generating and integrating information across multiple modes or types of data. These modes include text, images, audio, video or more.
Multimodal Generative AI systems are complex and involve several components to function effectively. Here are components of Multimodal Generative AI:
- Data Collection and Preprocessing
- Feature Extraction and Representation
- Multimodal Fusion
- Generative Models
- Cross-Modal Training
- Output Generation and Postprocessing
- Evaluation and Feedback
- Integration and Deployment
Multimodal Generative AI Capabilities
Here are few of the capabilities of multimodal Generative AI
- Text-to-Image Generation: Creating realistic images based on textual descriptions.
- Text-to-Video Generation: Producing videos from textual scripts or descriptions.
- Speech Synthesis: Converting text into natural-sounding speech.
- Image-to-Text Translation: Converting images into detailed textual descriptions.
- Text Summarization: Creating concise summaries of lengthy text documents.
- Audio-to-Text Transcription: Converting spoken language into written text.
- Multimodal Search: Combining inputs like text and images to refine search results.
- Personalized Content Generation: Creating customized content based on user preferences and inputs across various modalities.
- Language Translation with Contextual Understanding: Translating text while maintaining context from accompanying images or audio.
Benefits of Multimodal Generative AI
Increased efficiency - Unlike single modal AI systems, Multimodal GenAI understands and interprets multiple data types which leads to more accurate and relevant responses.
Enhanced Content Creation – Multimodal GenAI enables complex and rich content generation as it can integrate various data types (text, images, audio, video).
Personalization – Capable of creating content based on individual user preferences and inputs. Improved User Experience – Applications can offer more engaging experiences by combining different modalities.
Cross-Modal Insights – Multimodal Generative AI provides deeper and thorough insights as it integrates data from multiple modalities. Also helps in making more informed decisions by considering multiple data sources.
Conclusion
Multi-modal AI enables seamless integration and generation of diverse types of data such as text, images, audio and video improving user experience. It offers highly personalized experience and delivers rich user interactions across the fields including finance, healthcare, education and more. With GenAI multimodal capabilities, multiple modalities are not just integrated but can also generate multiple types of data. With the advancement in GenAI we will witness a lot of impact of GenAI multimodal AI on our daily lives which delivers richer and dynamic experience.